Long-exposure photo with light trails on a rooftop at dusk against a starry sky.
Energy and Environmental Implications of Artificial Intelligence
Key Observations
The rise of artificial intelligence (AI), especially generative AI models, is making energy consumption a big issue. For example, the International Energy Agency (IEA) says that using AI systems like ChatGPT can require up to 10 times more electricity than doing a regular Google search. From 2022 to 2026, the energy used by data centers, cryptocurrencies, and AI is expected to be about the same as what countries like Sweden or Germany use in a year. AI’s carbon footprint is also a concern. Training some models, like BLOOM, can produce as much greenhouse gas as 10 times the yearly emissions of an average French person. Reducing AI's energy use is a tough problem, and we need to be careful about how much and where we use it.
The Growing Energy Demands of AI
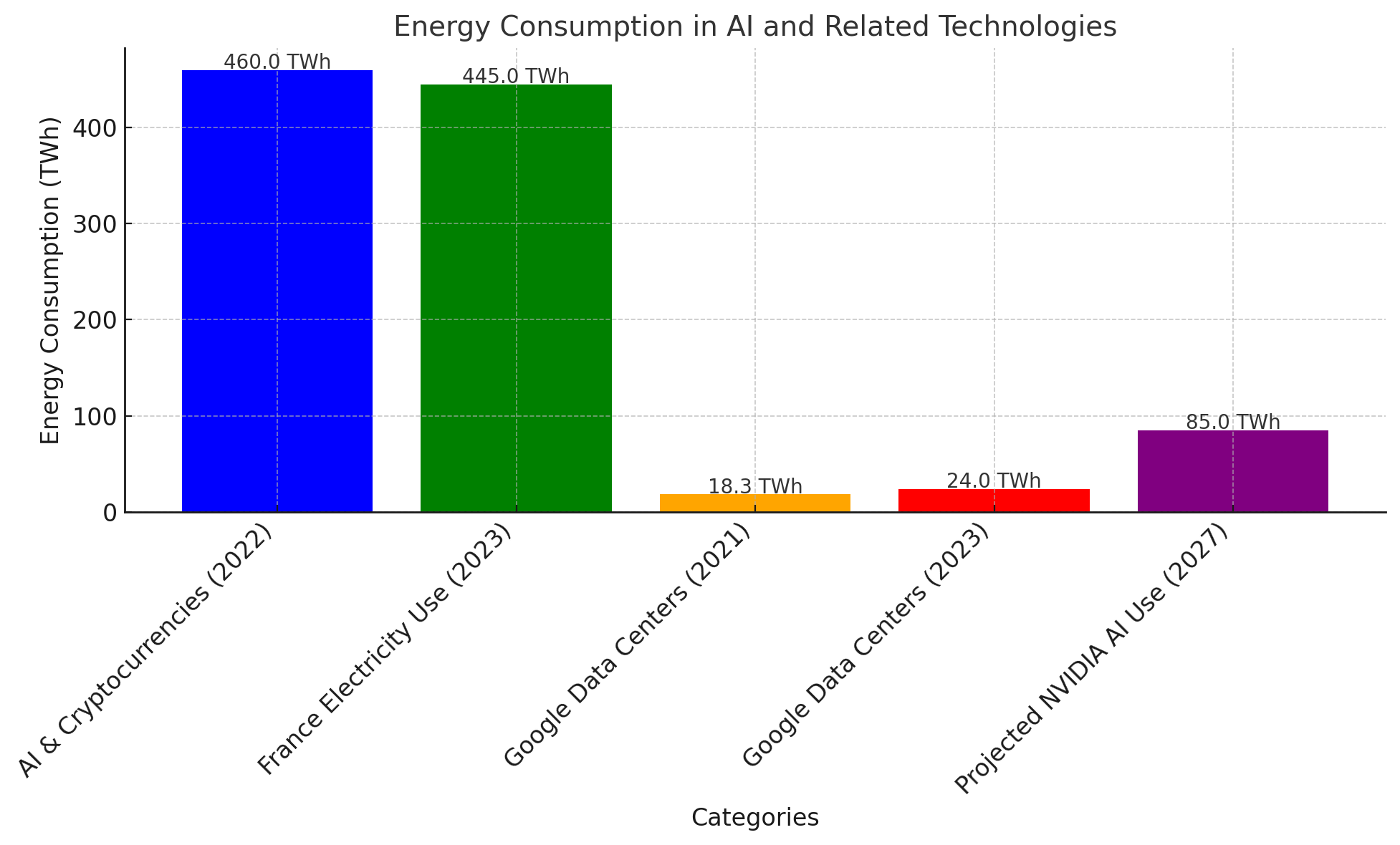
AI is being used in all kinds of areas, like healthcare, digital platforms, infrastructure, and transportation. It’s basically about computers doing tasks that usually need human judgment, but running AI takes a lot of energy, especially through data centers. In 2022, there were more than 8,000 data centers globally. The U.S. has about 33% of them, Europe has 16%, and China has close to 10%. Together with cryptocurrencies, AI used up about 2% of the world’s electricity in 2022—around 460 terawatt-hours (TWh), which is similar to France’s electricity use that same year (445 TWh).
Insufficient Data on AI Energy Use
It’s hard to figure out exactly how much energy AI uses because big tech companies don’t share enough details. Google, for example, said in 2022 that less than 15% of its energy use came from machine learning, which was no longer available beyond 2022. In 2023, Google data centers consumed 24 TWh of electricity-an increase from 18.3 TWh in 2021. Some researchers, such as Alex de Vries, estimate energy use for AI via an indirect measure; for example, using hardware sales. From NVIDIA's large share of the AI server market, de Vries thinks that AI would have used between 5.7 and 8.9 TWh of electricity in 2023. This is not to mention that the share of the total energy volume of data centers will be considerable.
The Impact of Generative AI
Generative AI models like ChatGPT, Bing Chat, and DALL-E have been expanding fast, which has made energy demands shoot up. These models need a lot of computational power both during training and when they’re being used (inference). In the past, training used up the most energy, but now inference is the bigger issue, accounting for 60–70% of the energy use. By 2027, de Vries estimates that NVIDIA servers for AI could use 85 to 134 TWh annually. To put that in perspective, if every Google search (about 9 billion per day) were replaced by ChatGPT queries, global electricity use would go up by 10 TWh a year—about the same as Ireland’s electricity consumption.
AI's Carbon Footprint and Sustainability Challenges
AI's use of energy creates an inevitable rise in their carbon footprint. For example, few advanced AI like BLOOM contribute to the generation of a very large amount of greenhouse gases. BLOOM has been known to generate 50 tons of CO₂ comparable to the greenhouse gas emissions of an average young person in France in only one training session. And it’s not just training—the devices people use to interact with AI also add to the emissions. These terminals can account for 25–45% of an AI model’s total carbon footprint.
Tech companies are trying to go carbon neutral, but it’s hard to keep up. Even though they invest in renewable energy and more efficient systems, the electricity demand from data centers keeps growing faster than clean energy production. For example, Google’s emissions went up by 37% from 2022 to 2023, showing how tough it is to balance AI’s energy needs with environmental goals.
The Path Forward: Moderation and Strategic Deployment
Reducing AI’s environmental impact requires a combination of solutions. These include making hardware and models more efficient, improving infrastructure, and moving data centers to areas with cleaner energy. But even these steps have limits. For instance, creating new hardware in the industry can cause emissions to be generated, and enhancing efficiency that causes a rise can,rebound-effects arising.
But according to de Vries, "It has been safely said that artificial intelligence must be judiciously used, with major applications being limited as much as possible to things like tangible benefits for the world." Otherwise, at the rate AI is growing, energy problems will get worse and slow down decarbonization.